Welcome!
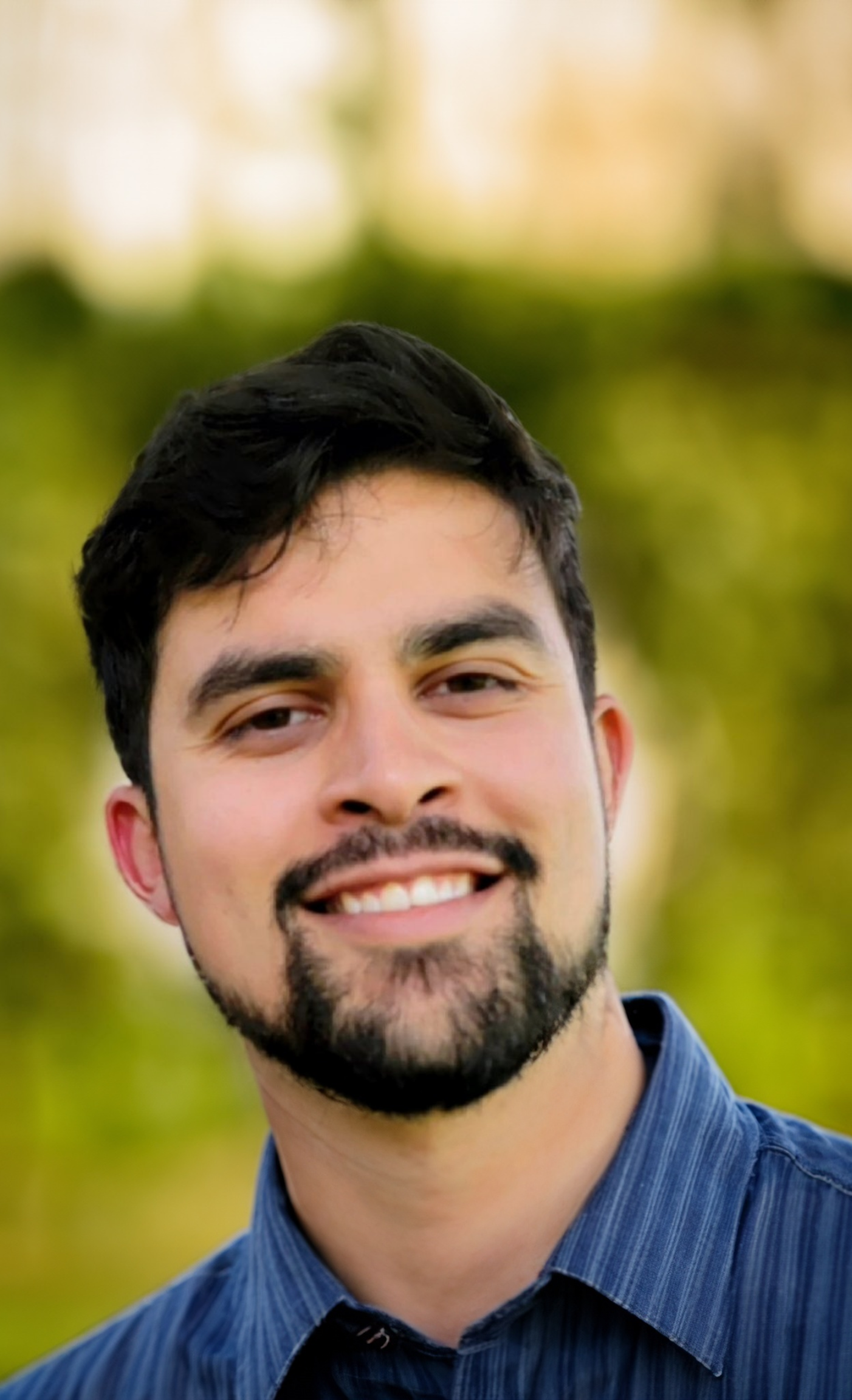
I am currently a machine learning engineer at Adobe in San Jose, CA.
My areas of interest include causal inference, causal discovery, and generally the intersection of machine learning and statistics.
Previously, I was a PhD student in statistics at UCLA (2017-22). My dissertation advisors were Qing Zhou and Oscar Madrid Padilla.
Publications
- G Ruiz, OH Madrid-Padilla, Q Zhou. “Sequentially learning the topological ordering of directed acyclic graphs with likelihood ratio scores.” Transactions on Machine Learning Research, 2022. [Link, R Package, Review Slides].
- M Burkhart, G Ruiz. “Neuroevolutionary feature representations for causal inference.” International Conference on Computational Science, 2022. [Link, Slides]
- OA Vsevolozhskaya, G Ruiz, DV Zaykin. “Bayesian prediction intervals for assessing P-value variability in prospective replication studies.” Translational Psychiatry, 2017. [Link]
- OA Vsevolozhskaya, CL Kuo, G Ruiz, L Diatchenko, DV Zaykin. “The more you test, the more you find: The smallest Pvalues become increasingly enriched with real findings as more tests are conducted.” Genetic Epidemiology, 2017. [Link]
Preprints and Working Papers
Causal Inference
- G Ruiz. “Sample size planning for conditional counterfactual mean estimation with a K-armed randomized experiment.” arXiv Preprint, 2024. [Link]
- G Ruiz, OH Madrid-Padilla. “Non-asymptotic confidence bands on the probability an individual benefits from treatment (PIBT).” arXiv Preprint, 2022. [Link, Poster]
- OH Madrid-Padilla, P Ding, Y Chen, G Ruiz. “A causal fused lasso for interpretable heterogeneous treatment effects.” arXiv Preprint, 2021. [Link]
Structural Equation Modeling
- G Ruiz, OH Madrid-Padilla, Q Zhou. “Scalable causal discovery with tunable exponential or power law tail decay and family-wise error control.” Working Paper, 2023+.
Grants:
NSF GRFP DGE-1650604
Work Experience
- I received my B.S. in statistics from University of Calfornia, Riverside in 2017 and had the pleasure of working there with Dr. Subir Ghosh through the MARC U program.
- I had the pleasure of working for Dmitri Zaykin at the National Institute of Environmental Health Sciences in Research Triangle Park, North Carolina in Summer 2016 on simulation work related to multiple hypothesis testing in genetics.
- In Summer 2017, I worked at Draper Laboratory in Cambridge, MA with an engineering team in the Perception and Localization group thanks to the GEM Consortium Fellowship.
- As a repeat data scientist intern at Adobe Inc., I worked on churn classification models and causal modeling in Summers 2019 and 2021, respectively.
Teaching
I was a Teaching Assistant at UCLA for Statistics 10: Introductory Statistics (6x), Statistics 100A: Probability with Texas Hold ‘Em Examples (1x), Statistics 100C: Linear Models (1x), and Statistics 200C: High Dimensional Statistics (1x).
Miscellaneuous
LinkedIn